AI in Performance Management: Strategic Use, Not Hype
Explore how AI can support—not replace—performance management. Benefits, risks, use cases, and prompts for smarter, more human-centered reviews.
Let’s talk about AI in performance management—critically and strategically. No buzzwords. No inflated promises. Just a grounded look at how generative AI can really integrate into performance management practices, and where we need to stay cautious.
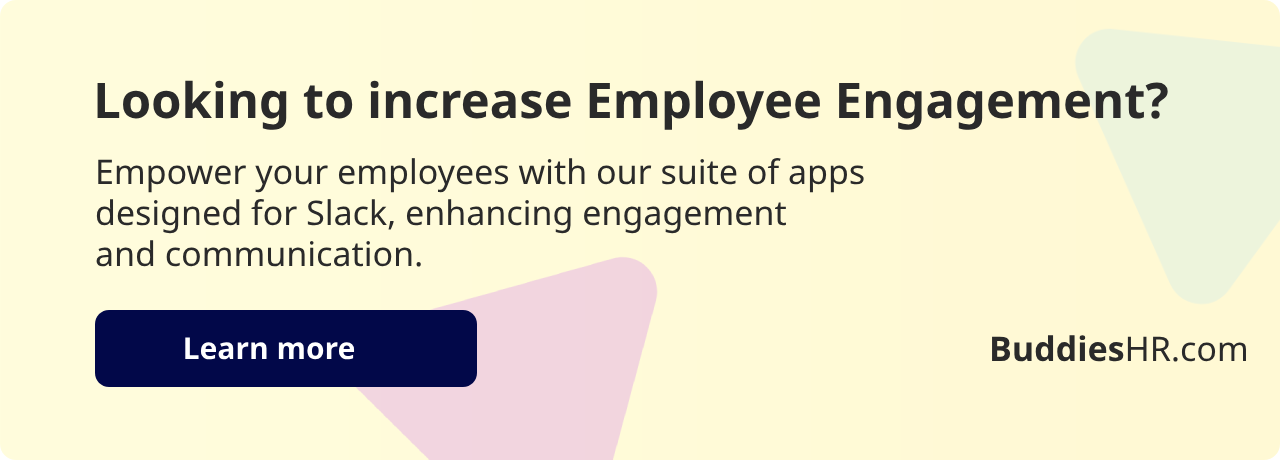
What Do We Mean by “AI in Performance Management”?
When we talk about applying generative AI here, we’re referring to tools that can:
- Draft performance reviews based on peer/manager feedback
- Identify high and low performers using behavioral or productivity data
- Suggest tailored development plans based on individual needs
- Monitor sentiment and feedback from check-ins or pulse surveys
- Send nudges to managers (e.g., “You haven’t given feedback to Sarah in 3 months”)
If you’re nodding along to the scope above but haven’t started integrating AI into your process yet—read on before slotting it into next quarter’s OKRs.
Potential Benefits (Let’s Be Real About Them)
- Time Efficiency
AI can help managers draft reviews and aggregate feedback faster. - Consistency and Objectivity
Standardized insights reduce human bias (like recency or halo/horns effect). - Real-Time Nudging
Surfaces issues before they become problems—no more waiting for annual reviews. - Personalized Development Plans
AI can suggest growth opportunities based on role, goals, and strengths. - Scalability
Works just as well for teams of 50 or 5,000.
But before we get carried away...
The Real Challenges (Worth Debating)
- Bias Isn’t Eliminated—It’s Shifted
AI learns from historical data, which can reflect and amplify existing biases. - Loss of Human Judgment
Can an algorithm recognize when someone underperformed due to a personal crisis—or understand the quiet impact of a mentor? - Trust and Transparency
Employees may distrust AI-generated feedback, especially if it's opaque. Psychological safety could take a hit. - Over-Reliance on Quantifiable Inputs
Not everything important is measurable—collaboration, emotional labor, resilience. - Manager Skill Decay
If AI does the “hard parts,” will managers stop practicing essential skills like giving feedback and handling tough conversations?
So… What’s the Sweet Spot?
AI should augment, not replace. Think of it as a co-pilot:
- Like Grammarly for performance reviews—suggestions, not conclusions
- A prompt for deeper conversations, not a substitute for them
- A calibration tool that offers insight, not a final verdict
The Performance Management Cycle + AI Touchpoints
Let’s explore how AI could fit into each stage of the cycle, with prompts and use cases—plus the risks to watch for.
1. Gathering Performance Evidence
Use Case: Summarizing 360° feedback
AI Prompt:
“Summarize the following peer and manager feedback into three key strengths and three areas for development for [employee name], using specific examples where possible. Tone: professional and balanced.”
Challenge:
Do we lose nuance when we summarize too much?
2. Writing Performance Reviews
Use Case: Drafting reviews based on goals and reflections
AI Prompt:
“Using these goals and self-reflections from [employee name], generate a performance review highlighting achievements, development areas, and recommendations. Tone: supportive but constructive.”
Challenge:
Helpful time-saver—or gateway to thoughtless, generic reviews?
3. Performance Calibration
Use Case: Spotting patterns across teams
AI Prompt:
“Analyze performance ratings and written feedback across the Sales department. Highlight patterns of leniency or severity and inconsistencies between narratives and scores.”
Challenge:
Can we flag inconsistencies without undermining manager autonomy?
4. Creating Development Plans
Use Case: Suggesting tailored growth opportunities
AI Prompt:
“Based on [employee name]’s strength in stakeholder management and development area in strategic thinking, suggest three specific growth opportunities (projects, mentoring, courses).”
Challenge:
Are we delivering meaningful ideas or generic advice that lacks context?
5. Supporting Employee Self-Reflection
Use Case: Helping employees write meaningful self-reviews
AI Prompt:
“Help me write a self-reflection based on these goals: [list goals]. I want to highlight impact, growth, and areas for development. Tone: confident but humble.”
Challenge:
Is this encouraging thoughtful reflection—or performative polishing?
Bonus: AI-Powered Calibration Debrief
Use Case: Cross-department calibration insights
AI Output Example:
"The Commercial team shows a higher frequency of 'Exceeds Expectations' ratings compared to Product, despite similar outcomes. This may suggest rating inflation—consider a calibration conversation."
Challenge:
Would your team trust this kind of insight—or resist it?
Final thoughts
AI in performance management isn’t about replacing human judgment—it’s about enhancing it. If used wisely, it can bring clarity, consistency, and efficiency. But if we lean too hard on automation, we risk losing the human insight that makes performance conversations meaningful. The key is to stay intentional, stay curious, and keep the people at the center.
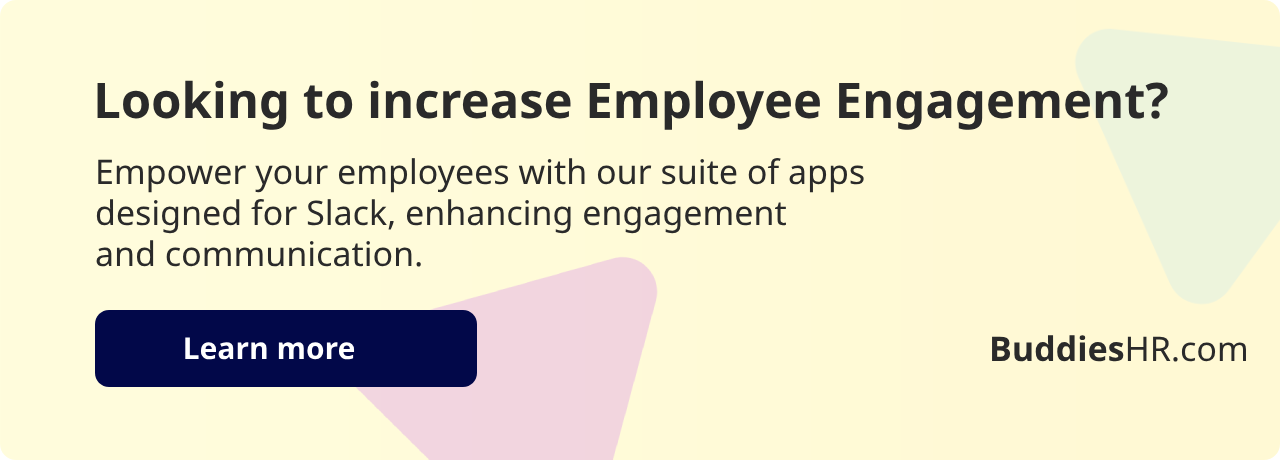